The Trend You Should Know about Edge AI Chip Technology
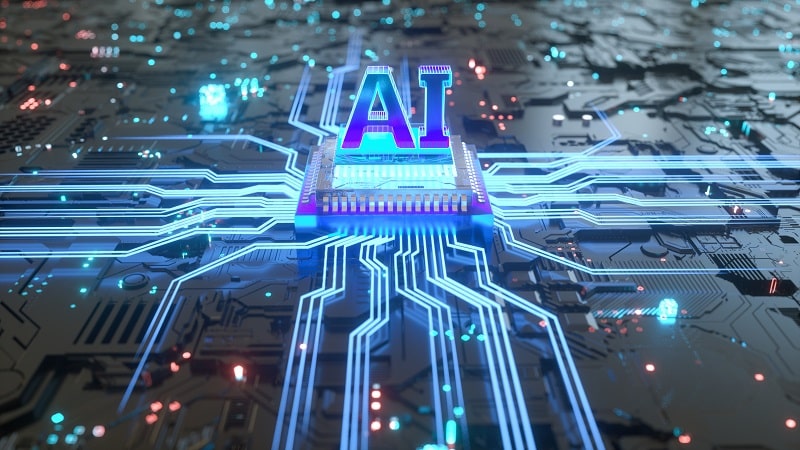
More and more people pay attention to Edge AI chips, meanwhile numbers of well-known manufacturers have been devoted to developing their own AI chips. In this article, we are going to have deeper discussion on the propositions of the Edge AI chips and its concepts.
Since Google released the Edge TPU chip last year, the AI chips for Edge computing have got people’s attention. Actually, lots of trained AI models should be installed on the front end and execute the real-time operations on the front end, e.g. real-time facial recognition for access control, real-time traffic conditions, etc. The execution is on the front end so that the performance is expected to be efficient, and low-power. That is why AI accelerator chip (Edge AI chip) is required.
The Edge AI chip market is forecast to grow so that numbers of chip manufacturers have decided to invest in this market, including traditional chip manufacturers, like Intel, Renesas, and newly established suppliers, such as AIStorm, Hailo, Flex Logic, Efinix, Cornami, Cambricon Tech, Xnor, etc. Edge AI chips have been so popular, yet the proposition might be still confusing. We are going to have further discussion on it.
Where is the Edge AI chip used?
Edge AI chip is generally used in IoT gateway or a sensor node that has better computing capabilities and sufficient hardware resources. Edge AI chip is rarely placed in smart phones because of the limited height and space. If the developers need to have hardware acceleration for AI computing, they will choose to design AI acceleration circuit in Application Processor in smart phone or use the SoC with AI function. Developers rarely use an independent accelerator chip in a smart phone.
Edge AI is One of Inference Chips
Since AI technologies became popular again in 2016, numbers of trained models need to be executed. To optimize the inference execution, AI acceleration specifically designed for inference is launched. Edge AI is just one of the inference chips but not the only one. Now there is also a type of inference chip specifically used in data center, such as NVIDIA Tesla T4 accelerator. Its power consumption is about 70 watt, so generally it’s not suitable for Edge application.
Last November, AWS announced that Inferentia chip will be released in 2019, which is also an AI inference chip suitable for data center. FPGA chip manufacturer, Xillinx, also released Alveo, which is AI inference FPGA accelerator card for data center as well. Additionally, an Israel startup, Habana Labs, also released Goya HL-1000 accelerator card, too.
Some inference chips for data center are placed at front end, such as Lenovo Edge Server: SE350, a server (IoT gateway) can be installed on site. NVIDIA Tesla T4 can be installed in SE350, but the power supply should be sufficient.
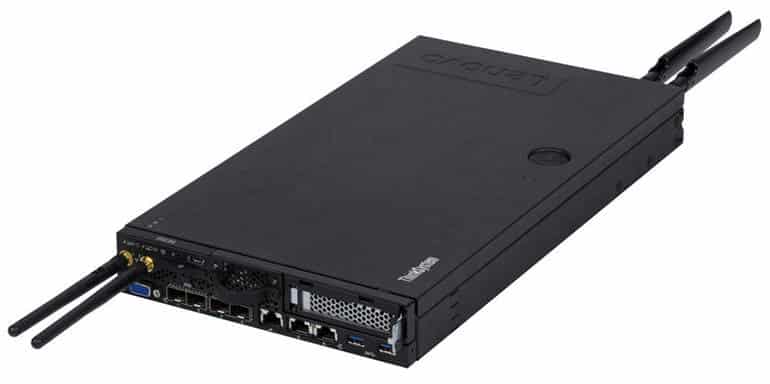
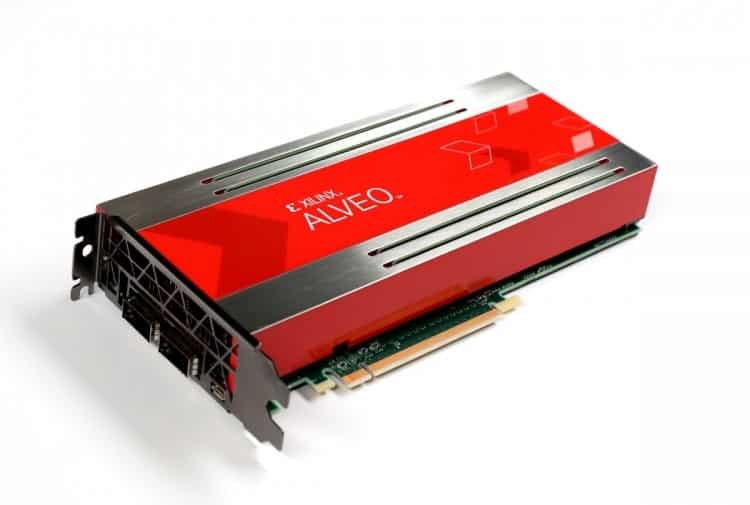
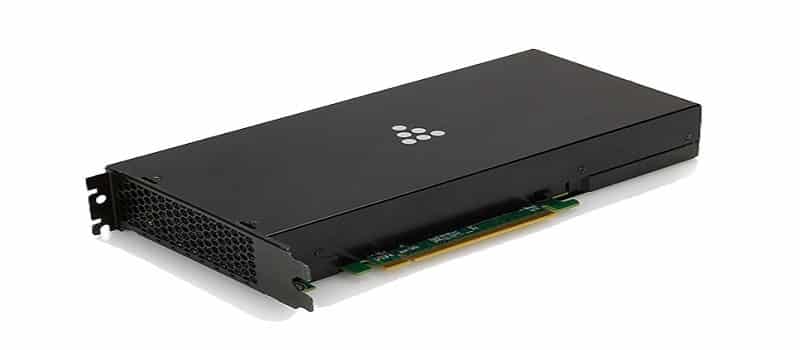
Another exception is the Edge AI chip for automotive industry. There are energy storage bottles in vehicles, so the power is greater than handheld devices, like smart phone. However, it’s still weaker than the plug of alternating current household electricity. The vehicle allows to use more electricity to execute the AI inference on Edge end. It is one of the Edge AI chips as well, yet the AI chip consumes more power.
There is no clear difference among Edge AI chips for automotive industry, data center AI inference chips, and Edge AI chips, but I suggest these chips can be categorized by power consumption on subsystem board, by 10 watts, and even lower 5 watts, 1 watt, etc.
Reduced Precision, Mixed Precision, and Positive Integer
At training stage, the AI computing will be executed with 32-bit or 16-bit floating points; at inference stage, it will tend to be computed with integers, such as 32-bit or 16-bit integers due to different purposes. Also, the AI computing will be executed even with reduced precision, e.g. 8-bit, 4-bit. For example, Google Edge TPU supports INT16, INT8, the integer inference computing but does not support floating points.
Besides reduced precision, mixed precision is also needed. That means the computing is executed with integers and floating points simultaneously. For example, AWS Inferentia computes INT8 and FP16 at the same time. Another case is to use mixed precision floating points. For example, NVIDIA Tesla T4 uses FP16 and FP32 together. Some even use positive integers only and do not use any negative integers; for example, Habana Labs HL-1000 supports positive integers, UINT8/16/32. These requirements can be applied to the inference chips in data center and Edge AI chips.
Package Size and TOPS/Watt
For each Edge AI chip, the energy and available space within the device is all limited, so the chip manufacturers focus on the performance per watt (TOPS/Watt or Tflops/Watt), and the chip package size, volume, and size. That is why some manufacturers would like to put Edge AI chip and coins in the same photo to show how tiny the chip is.
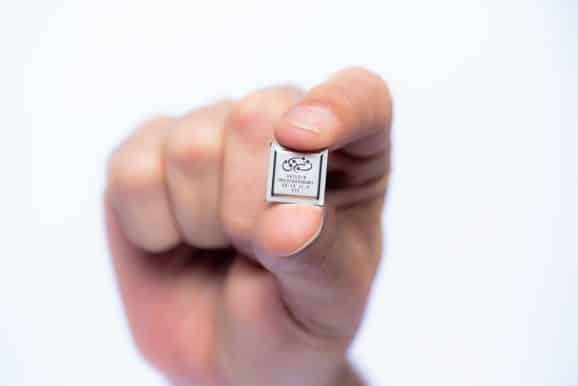
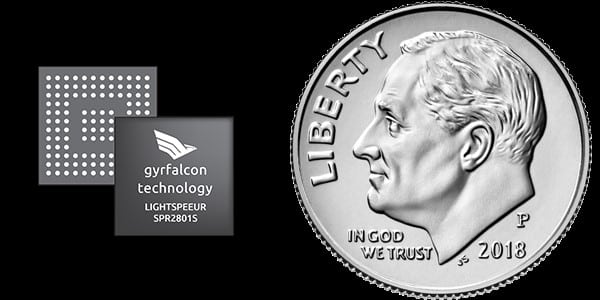
In terms of the performance per watt, the startup “Hailo”, has the chip “Hailo-8” can reach 26TOPS under INT8 precision. Japanese manufactures, Renesas, announced that they will release a new R-Car series SoC with 5TOPS per watt. Also, Intel announced that they will launch new EyeQ5 chip with 24TOPS @10W. These are all the evidences that these chips can reach high performance under a specific watt.
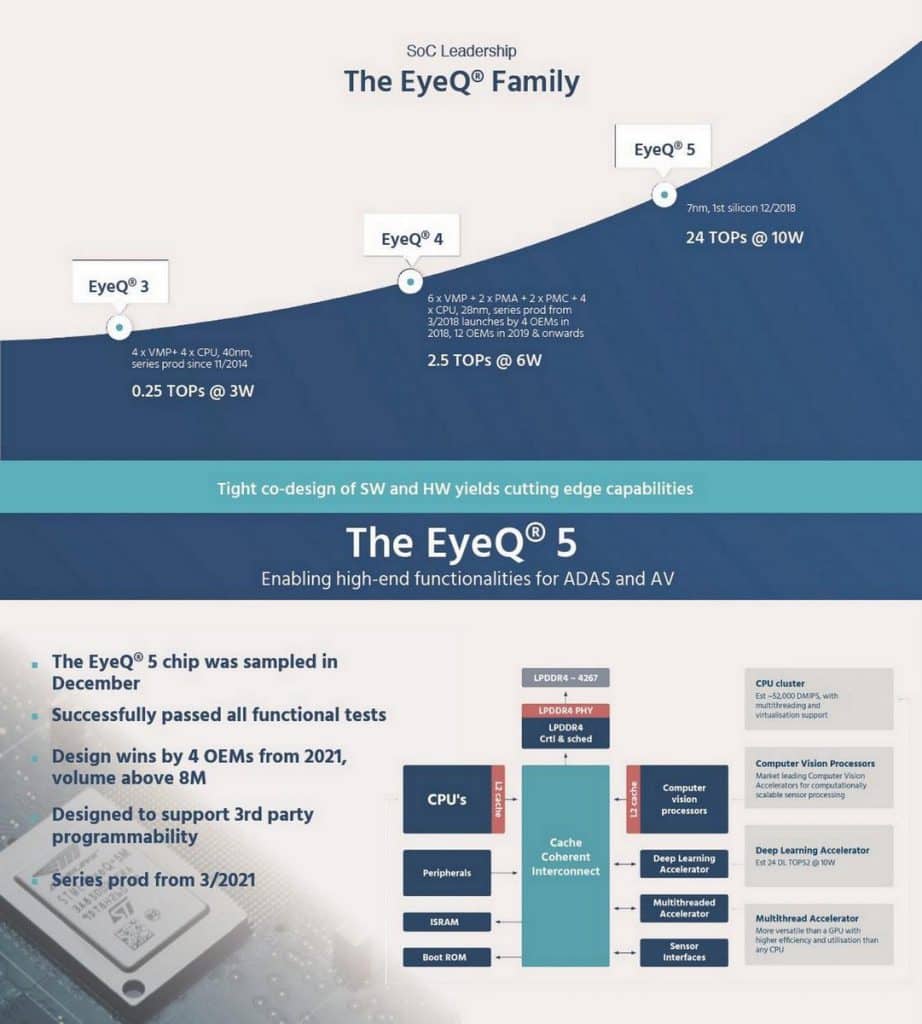
(The original Chinese version of this article was written by Hsiang-yang Lu and published on MakerPRO)
Sign up to become TechDesign member and get the first-hand supply chain news.
Keep on working, great job!
Thanks for reaching out. We’ll keep it up!